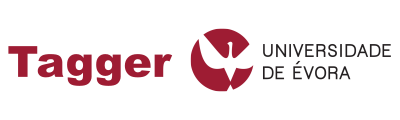
File processing
Input format: Input files must be in .txt FORMAT with UTF-8 ENCODING and contain PORTUGUESE TEXT. Input files and folders can also be compressed to the .zip format.
Privacy: The input file you upload and the respective output files will be automatically deleted from our computer after being processed and the result downloaded by you. No copies of your files will be retained after your use of this service.
Email address validation
Loading...
The size of your input file is large and its processing may take some time.
To receive by email an URL from which to download your processed file, please copy the code displayed below into the field "Subject:" of an email message (with the message body empty) and send it to request@portulanclarin.net
To proceed, please send an email to request@portulanclarin.net with the following code in the "Subject" field:
To: | request@portulanclarin.net |
|
Subject: |
|
The communication with the server cannot be established. Please try again later.
We are sorry but an unexpected error has occurred. Please try again later.
The code has expired. Please click the button below to get a new code.
For enhanced security, a new code has to be validated. Please click the button below to get a new code.
Privacy: After we reply to you with the URL for download, your email address is automatically deleted from our records.
Designing your own experiment with a Jupyter Notebook
A Jupyter notebook (hereafter just notebook, for short) is a type of document that contains executable code interspersed with visualizations of code execution results and narrative text.
Below we provide an example notebook which you may use as a starting point for designing your own experiments using language resources offered by PORTULAN CLARIN.
Pre-requisites
To execute this notebook, you need an access key you can obtain by clicking the button below. A key is valid for 31 days. It allows to submit a total of 1 billion characters by means of requests with no more 4000 characters each. It allows to enter 100,000 requests, at a rate of no more than 200 requests per hour.
For other usage regimes, you should contact the helpdesk.
The input data sent to any PORTULAN CLARIN web service and the respective output will be automatically deleted from our computers after being processed. However, when running a notebook on an external service, such as the ones suggested below, you should take their data privacy policies into consideration.
Running the notebook
You have three options to run the notebook presented below:
- Run on Binder — The Binder Project is funded by a 501c3 non-profit
organization and is described in detail in the following paper:
Jupyter et al., "Binder 2.0 - Reproducible, Interactive, Sharable Environments for Science at Scale."
Proceedings of the 17th Python in Science Conference. 2018. doi://10.25080/Majora-4af1f417-011 - Run on Google Colab — Google Colaboratory is a free-to-use product from Google Research.
- Download the notebook from our public Github repository and run it on your computer.
This is a more advanced option, which requires you to install Python 3 and Jupyter on your computer. For anyone without prior experience setting up a Python development environment, we strongly recommend one of the two options above.
This is only a preview of the notebook. To run it, please choose one of the following options:
Using UEvora Tagger to annotate a text from the BDCamões corpus¶
This is an example notebook that illustrates how you can use the UEvora Tagger web service to annotate a sample text from the BDCamões corpus (the full corpus is available from the PORTULAN CLARIN repository).
Before you run this example, replace access_key_goes_here
by your webservice access key, below:
UEVORA_TAGGER_WS_API_KEY = 'access_key_goes_here'
UEVORA_TAGGER_WS_API_URL = 'https://portulanclarin.net/workbench/uevora-tagger/api/'
Importing required Python modules¶
The next cell will take care of installing the requests
and matplotlib
packages,
if not already installed, and make them available to use in this notebook.
try:
import requests
except:
!pip3 install requests
import requests
try:
import matplotlib.pyplot as plt
except:
!pip3 install matplotlib
import matplotlib.pyplot as plt
import collections
Wrapping the complexities of the JSON-RPC API in a simple, easy to use function¶
The WSException
class defined below, will be used later to identify errors
from the webservice.
class WSException(Exception):
'Webservice Exception'
def __init__(self, errordata):
"errordata is a dict returned by the webservice with details about the error"
super().__init__(self)
assert isinstance(errordata, dict)
self.message = errordata["message"]
# see https://json-rpc.readthedocs.io/en/latest/exceptions.html for more info
# about JSON-RPC error codes
if -32099 <= errordata["code"] <= -32000: # Server Error
if errordata["data"]["type"] == "WebServiceException":
self.message += f": {errordata['data']['message']}"
else:
self.message += f": {errordata['data']!r}"
def __str__(self):
return self.message
The next function invokes the UEvora Tagger webservice through its public JSON-RPC API.
def tag(text, format):
'''
Arguments
text: a string with a maximum of 4000 characters, Portuguese text, with
the input to be processed
format: either 'column' or 'JSON'
Returns a string with the output according to specification in
https://portulanclarin.net/workbench/uevora-tagger/
Raises a WSException if an error occurs.
'''
request_data = {
'method': 'tag',
'jsonrpc': '2.0',
'id': 0,
'params': {
'text': text,
'format': format,
'key': UEVORA_TAGGER_WS_API_KEY,
},
}
request = requests.post(UEVORA_TAGGER_WS_API_URL, json=request_data)
response_data = request.json()
if "error" in response_data:
raise WSException(response_data["error"])
else:
return response_data["result"]
Let us test the function we just defined:
text = '''Esta frase serve para testar o funcionamento do tagger. Esta outra
frase faz o mesmo.'''
# The column annotation format is a simple format where each line contains
# one token and its part of speech tag, separated by a tab.
# An empty line marks the end of a sentence.
result = tag(text, format="column")
print(result)
Esta PRO frase N serve V para PREP testar V o DET funcionamento N do PREPXDET tagger PREP . PU Esta PRO outra PRO frase N faz V o DET mesmo ADV . PU
The JSON output format¶
The JSON format (which we obtain by passing format="JSON"
into the annotate
function) is more
convenient when we need to further process the annotations, because each abstraction is mapped
directly into a Python native object (lists, dicts, strings, etc) as follows:
- The returned object is a
list
, where each element corresponds to a paragraph of the given text; - In turn, each paragraph is a
list
where each element represents a sentence; - Each sentence is a
list
where each element represents a token; - Each token is represented as a
list
with two elements: the token itself and the corresponding part-of-speech tag.
annotated_text = tag(text, format="JSON")
for pnum, paragraph in enumerate(annotated_text, start=1): # enumerate paragraphs in text, starting at 1
print(f"paragraph {pnum}:")
for snum, sentence in enumerate(paragraph, start=1): # enumerate sentences in paragraph, starting at 1
print(f" sentence {snum}:")
for tnum, token in enumerate(sentence, start=1): # enumerate tokens in sentence, starting at 1
print(f" token {tnum}: {token!r}") # print a token representation
paragraph 1: sentence 1: token 1: ['Esta', 'PRO'] token 2: ['frase', 'N'] token 3: ['serve', 'V'] token 4: ['para', 'PREP'] token 5: ['testar', 'V'] token 6: ['o', 'DET'] token 7: ['funcionamento', 'N'] token 8: ['do', 'PREPXDET'] token 9: ['tagger', 'PFX'] token 10: ['.', 'PU'] sentence 2: token 1: ['Esta', 'PRO'] token 2: ['outra', 'PRO'] token 3: ['frase', 'N'] token 4: ['faz', 'V'] token 5: ['o', 'DET'] token 6: ['mesmo', 'ADV'] token 7: ['.', 'PU']
Downloading and preparing our working text¶
In the next code cell, we will download a copy of the book "Viagens na minha terra" and prepare it to be used as our working text.
# A plain text version of this book is available from our Gitbub repository:
sample_text_url = "https://github.com/portulanclarin/jupyter-notebooks/raw/main/sample-data/viagensnaminhaterra.txt"
req = requests.get(sample_text_url)
sample_text_lines = req.text.splitlines()
num_lines = len(sample_text_lines)
print(f"The downloaded text contains {num_lines} lines")
# discard whitespace at beginning and end of each line:
sample_text_lines = [line.strip() for line in sample_text_lines]
# discard empty lines
sample_text_lines = [line for line in sample_text_lines if line]
# how many lines do we have left?
num_lines = len(sample_text_lines)
print(f"After discarding empty lines we are left with {num_lines} non-empty lines")
The downloaded text contains 2509 lines After discarding empty lines we are left with 2205 non-empty lines
Annotating with the UEvora Tagger web service¶
There is a limit on the number of web service requests per hour that can be made in association with any given key. Thus, we should send as much text as possible in each request while also conforming with the 4000 characters per request limit.
To this end, the following function slices our text into chunks smaller than 4K:
def slice_into_chunks(lines, max_chunk_size=4000):
chunk, chunk_size = [], 0
for lnum, line in enumerate(lines, start=1):
if (chunk_size + len(line)) <= max_chunk_size:
chunk.append(line)
chunk_size += len(line) + 1
# the + 1 above is for the newline character terminating each line
else:
yield "\n".join(chunk)
if len(line) > max_chunk_size:
print(f"line {lnum} is longer than 4000 characters; truncating")
line = line[:4000]
chunk, chunk_size = [line], len(line) + 1
if chunk:
yield "\n".join(chunk)
Next, we will apply slice_into_chunks
to the sample text to get the chunks to be annotated.
chunks = list(slice_into_chunks(sample_text_lines))
annotated_text = [] # annotated paragraphs will be stored here
chunks_processed = 0 # this variable keeps track of which chunks have been processed already
print(f"There are {len(chunks)} chunks to be annotated")
There are 105 chunks to be annotated
Next, we will invoke annotate
on each chunk. Note: annotating each chunk with this tagger takes about 30 secs.
If we get an exception while annotating a chunk:
- check the exception message to determine what was the cause;
- if the maximum number of requests per hour has been exceeded, then wait some time before retrying;
- if a temporary error occurred in the webservice, try again later.
In any case, as long as the notebook is not shutdown or restarted, the text that has been annotated thus far is not lost, and re-running the following cell will pick up from the point where the exception occurred.
for cnum, chunk in enumerate(chunks[chunks_processed:], start=chunks_processed+1):
try:
annotated_text.extend(tag(chunk, format="JSON"))
chunks_processed = cnum
# print one dot for each annotated chunk to get some progress feedback
print(".", end="", flush=True)
except Exception as exc:
chunk_preview = chunk[:100] + "[...]" if len(chunk) > 100 else chunk
print(
f"\nError: annotation of chunk {cnum} failed ({exc}); chunk contents:\n\n{chunk_preview}\n\n"
)
break
.........................................................................................................
Let's create a pie chart with the most common part-of-speech tags¶
%matplotlib inline
tag_frequencies = collections.Counter(
tag
for paragraph in annotated_text
for sentence in paragraph
for token, tag in sentence
).most_common()
tags = [tag for tag, _ in tag_frequencies[:9]]
freqs = [freq for _, freq in tag_frequencies[:9]]
tags.append("other")
freqs.append(sum(freq for _, freq in tag_frequencies[10:]))
plt.rcParams['figure.figsize'] = [10, 10]
fig1, ax1 = plt.subplots()
ax1.pie(freqs, labels=tags, autopct='%1.1f%%', startangle=90)
ax1.axis('equal') # equal aspect ratio ensures that pie is drawn as a circle.
plt.show()
# To learn more about matplotlib visit https://matplotlib.org/
Getting the status of a webservice access key¶
def get_key_status():
'''Returns a string with the detailed status of the webservice access key'''
request_data = {
'method': 'key_status',
'jsonrpc': '2.0',
'id': 0,
'params': {
'key': UEVORA_TAGGER_WS_API_KEY,
},
}
request = requests.post(UEVORA_TAGGER_WS_API_URL, json=request_data)
response_data = request.json()
if "error" in response_data:
raise WSException(response_data["error"])
else:
return response_data["result"]
get_key_status()
{'requests_remaining': 99999870, 'chars_remaining': 999550140, 'expiry': '2030-01-10T00:00+00:00'}
Instructions to use this web service
The web service for this application is available at https://portulanclarin.net/workbench/uevora-tagger/api/.
Below you find an example of how to use this web service with Python 3.
This example resorts to the requests package. To install this package, run this command in the command line:
pip3 install requests
.
To use this web service, you need an access key you can obtain by clicking in the button below. A key is valid for 31 days. It allows to submit a total of 1 billion characters by means of requests with no more 4000 characters each. It allows to enter 100,000 requests, at a rate of no more than 200 requests per hour.
For other usage regimes, you should contact the helpdesk.
The input data and the respective output will be automatically deleted from our computer after being processed. No copies will be retained after your use of this service.
import json
import requests # to install this library, enter in your command line:
# pip3 install requests
# This is a simple example to illustrate how you can use the UEvora Tagger web service
# Requires: key is a string with your access key
# Requires: text is a string, UTF-8, with a maximum 4000 characters, Portuguese text, with
# the input to be processed
# Requires: format is a string, indicating the output format, which can be either
# 'column' or 'JSON'
# Ensures: output according to specification in https://portulanclarin.net/workbench/uevora-tagger/
# Ensures: dict with number of requests and characters input so far with the access key, and
# its date of expiry
key = 'access_key_goes_here' # before you run this example, replace access_key_goes_here by
# your access key
# this string can be replaced by your input
text = '''Esta frase serve para testar o funcionamento do tagger.
Esta outra frase faz o mesmo.'''
# To read input text from a file, uncomment this block
#inputFile = open("myInputFileName", "r", encoding="utf-8") # replace myInputFileName by
# the name of your file
#text = inputFile.read()
#inputFile.close()
format = 'column' # other possible value is 'JSON'
# Processing:
url = "https://portulanclarin.net/workbench/uevora-tagger/api/"
request_data = {
'method': 'tag',
'jsonrpc': '2.0',
'id': 0,
'params': {
'text': text,
'format': format,
'key': key,
},
}
request = requests.post(url, json=request_data)
response_data = request.json()
if "error" in response_data:
print("Error:", response_data["error"])
else:
print("Result:")
print(response_data["result"])
# To write output in a file, uncomment this block
#outputFile = open("myOutputFileName","w", encoding="utf-8") # replace myOutputFileName by
# the name of your file
#output = response_data["result"]
#outputFile.write(output)
#outputFile.close()
# Getting acess key status:
request_data = {
'method': 'key_status',
'jsonrpc': '2.0',
'id': 0,
'params': {
'key': key,
},
}
request = requests.post(url, json=request_data)
response_data = request.json()
if "error" in response_data:
print("Error:", response_data["error"])
else:
print("Key status:")
print(json.dumps(response_data["result"], indent=4))
Access key for the web service
This is your access key for this web service.
The following access key for this web service is already associated with .
This key is valid until and can be used to process requests or characters.
An email message has been sent into your address with the information above.
Email address validation
Loading...
To receive by email your access key for this webservice, please copy the code displayed below into the field "Subject" of an email message (with the message body empty) and send it to request@portulanclarin.net
To proceed, please send an email to request@portulanclarin.net with the following code in the "Subject" field:
To: | request@portulanclarin.net |
|
Subject: |
|
The communication with the server cannot be established. Please try again later.
We are sorry but an unexpected error has occurred. Please try again later.
The code has expired. Please click the button below to get a new code.
For enhanced security, a new code has to be validated. Please click the button below to get a new code.
Privacy: When your access key expires, your email address is automatically deleted from our records.
UEvora-Tagger's Documentation
UEvora-Tagger
UEvora Tagger is a freely available on-line service for tagging sentences written in Portuguese. This service was developed and is maintained at the University of Évora by the VISTA - Video, Image, Speech, and Text Analysis Group of the Department of Informatics.
Characteristics and evaluation
The UEvora Tagger is based on a machine learning methodology and it used a collection of previously classified Portuguese corpus as a training set.
Authorship
UEvora Tagger was developed by the VISTA@UE research group.
References
Please cite this reference if you use this tool in your research work:
- N. Miranda, R. Raminhos, P. Seabra, J. Sequeira, T. Gonçalves, and P. Quaresma, 2011. "Named entity recognition using machine learning techniques". In EPIA-11, 15th Portuguese Conference on Artificial Intelligence, Lisbon, PT, October 2011
Contacts
E-mail: 'pq' '@' 'uevora.pt'
License
No fee, attribution, all rights reserved, no redistribution, non commercial, no warranty, no liability, no endorsement, temporary, non exclusive, share alike.
The complete text of this license is here.